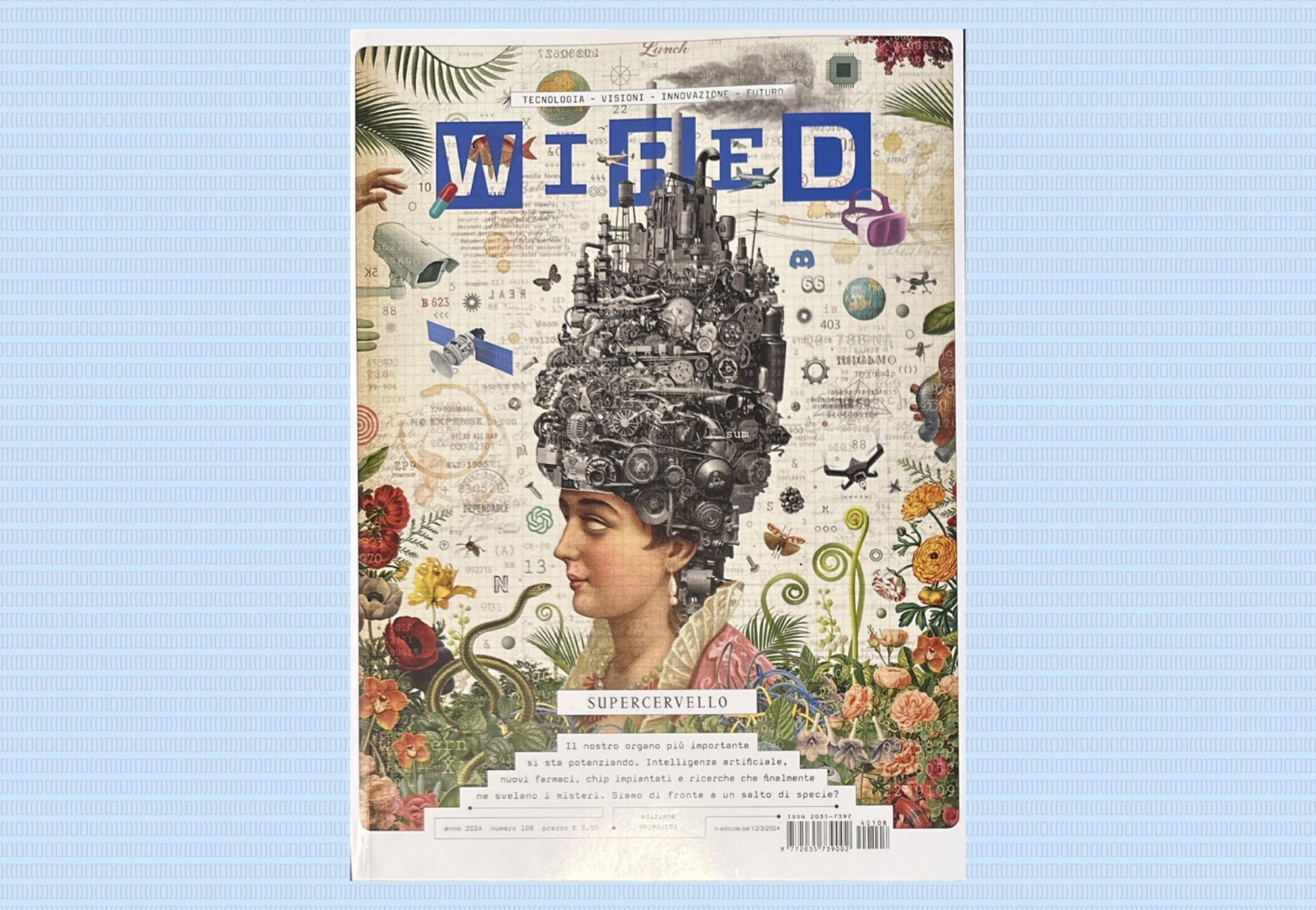
The “Humans in Humans Out” project aims at producing a generative synthetic dataset to evaluate large language models (LLMs) on reasoning patterns—both accurate and fallacious—that resemble human thinking. The project assesses whether LLMs replicate predictable human-like reasoning errors beyond what humans have seen or what is present in any extant dataset. The project builds on prior work that observed human-like fallacies in LLMs, particularly as these models expand in size and sophistication, and aims to uncover whether LLMs might converge toward common-sense reasoning patterns—even in erroneous judgments. Findings from this dataset could inform novel training strategies to enhance LLM performance on human-like inference, and shed light on what aspects of the human reasoning process are being approximated by LLMs trained on large human-generated data sets.
Download the ArXiv paper on preliminary work here.
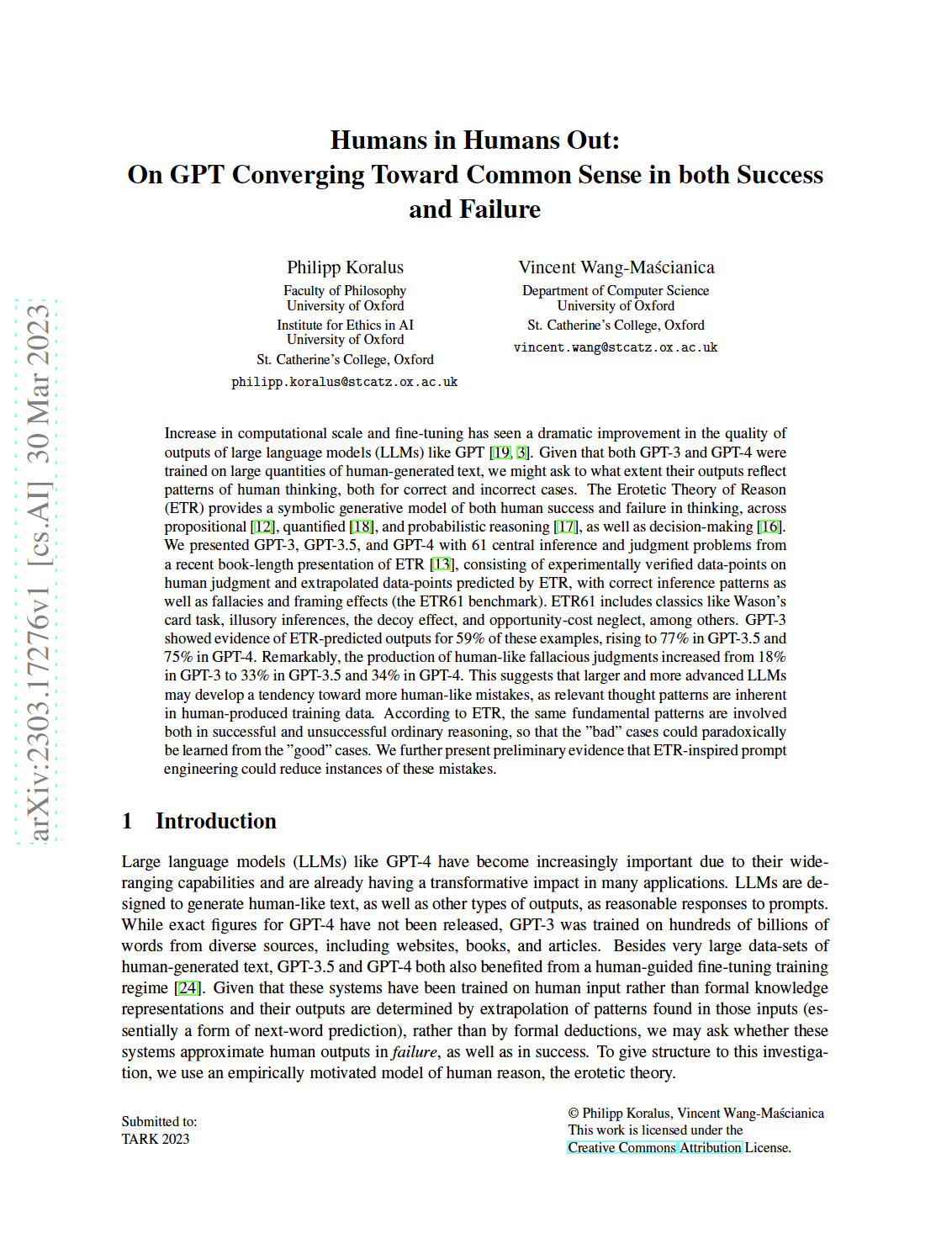